A novel single-cell RNA-sequencing approach and its applicability connecting genotype to phenotype in ageing disease
Shomroni O, Sitte M, Schmidt J, Parbin S, Ludewig F, Yigit G, Zelarayan LC, Streckfuss-Bömeke K, Wollnik B, Salinas G, 08.03.2022
Abstract
Single cell multi-omics analysis has the potential to yield a comprehensive understanding of the cellular events that underlie the basis of human diseases. The cardinal feature to access this information is the technology used for single-cell isolation, barcoding, and sequencing. Most currently used single-cell RNA-sequencing platforms have limitations in several areas including cell selection, documentation and library chemistry. In this study, we describe a novel high-throughput, full-length, single-cell RNA-sequencing approach that combines the CellenONE isolation and sorting system with the ICELL8 processing instrument. This method offers substantial improvements in single cell selection, documentation and capturing rate. Moreover, it allows the use of flexible chemistry for library preparations and the analysis of living or fixed cells, whole cells independent of sizing and morphology, as well as of nuclei. We applied this method to dermal fibroblasts derived from six patients with different segmental progeria syndromes and defined phenotype associated pathway signatures with variant associated expression modifiers. These results validate the applicability of our method to highlight genotype-expression relationships for molecular phenotyping of individual cells derived from human patients.
Shomroni, O., Sitte, M., Schmidt, J. et al. A novel single-cell RNA-sequencing approach and its applicability connecting genotype to phenotype in ageing disease. Sci Rep 12, 4091 (2022). https://doi.org/10.1038/s41598-022-07874-1
Publication: https://doi.org/10.1038/s41598-022-07874-1 Repository: https://www.ncbi.nlm.nih.gov/geo/query/acc.cgi?acc=GSE179211
Disclaimer
The publication A novel single-cell RNA-sequencing approach and its applicability connecting genotype to phenotype in ageing disease by Shomroni O, Sitte M, Schmidt J, Parbin S, Ludewig F, Yigit G, Zelarayan LC, Streckfuss-Bömeke K, Wollnik B, Salinas G is published under an open access license: https://creativecommons.org/licenses/by/4.0/. Permits non-commercial re-use, distribution, and reproduction in any medium, provided the original work is properly cited.
Curation by the MFGA team Relevant data sets presented in the publication have been identified. If possible, annotations (title, general information, conditions, processed tissue types and processed cell types) have been added based on information from the publication. Data tables and images that provide a good overview on the publication's findings on the data set have been extracted from the publication and/or supplement. If not stated otherwise, images are depicted with title and description exactly as in the publication. Tables have been adjusted to the MFGA table format. Conducted adjustments are explained in the detailed view of the tables. However, titles and descriptions have been adopted from the publication.
Data set 1: Cell cultures of eight primary dermal fibroblasts
Transcriptome: Single-cell RNA-Sequencing
Species
Species |
---|
Human |
Tissue Types
BRENDA tissue ontology | Maturity | Description | Species | Replicates |
---|---|---|---|---|
BTO_0004419: dermal fibroblast | Dermal fibroblasts are the major cell type in dermis and are commonly accepted as terminally differentiated cells. | Human | 8 |
Images

Figure 1: Analysis of transcriptional heterogeneity of dermal fibroblast derived from patients using the novel platform.
(a) t-SNE of the ICELL8 and CellenONE-ICELL8 experiments. (b) Circo plot showing connection of top 100 marker genes of the samples in both ICELL8 and CellenONE-ICELL8 platforms. (c) Unsupervised t-SNE of the CellenONE-ICELL8 approach. (d) t-SNE showing most prominent markers for different clusters. (e) Heatmap of rank scores of the top 30 markers in each unsupervised cluster, including enriched pathways.
Licensed under: https://creativecommons.org/licenses/by/4.0/
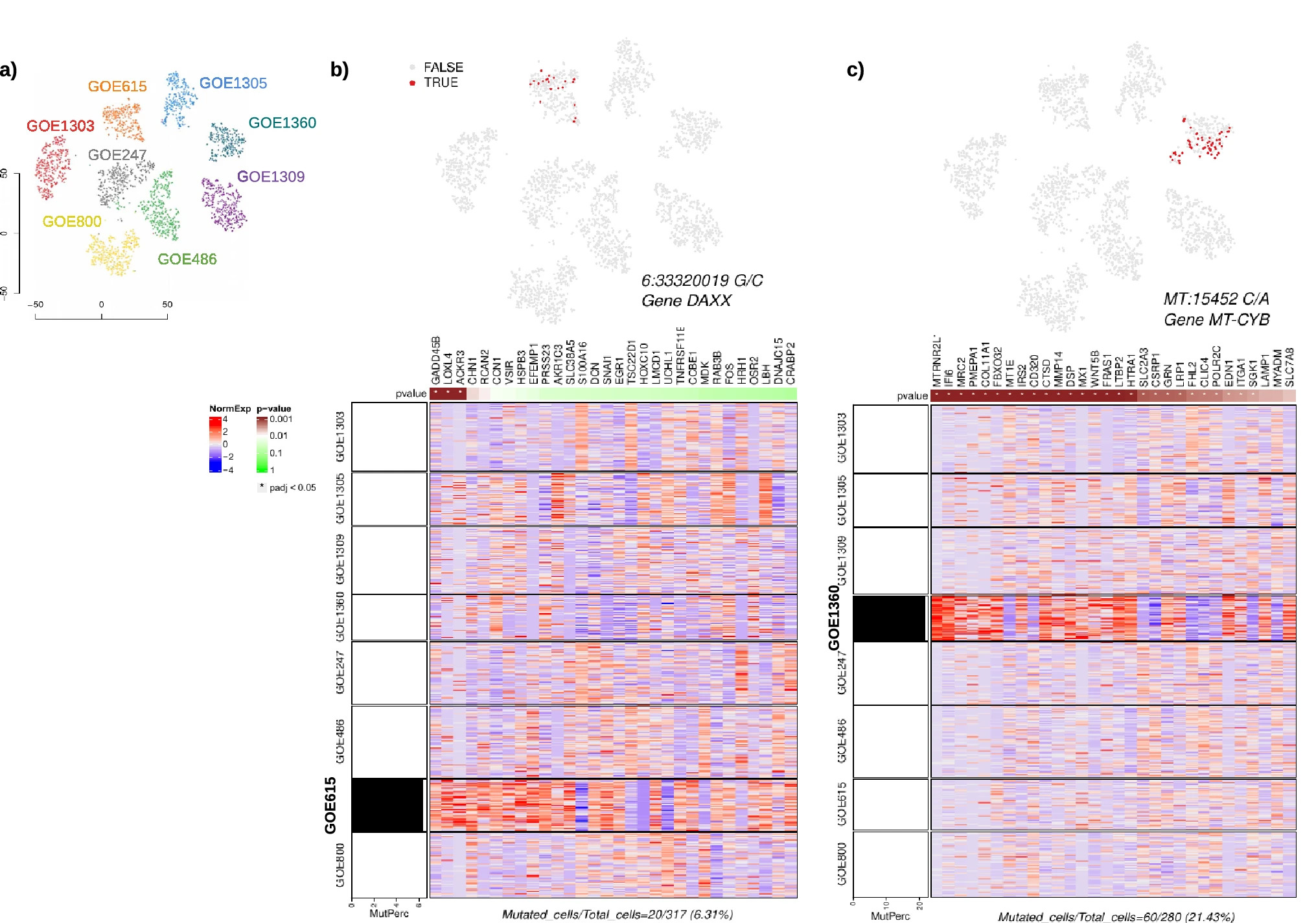
Figure 2: Analysis of mutational heterogeneity in dermal fibroblast derived patients.
(a) tSNE plot showing cells classified according to their sample affiliation. (b) t-SNE projection showing non-uniformly distributed DAXXA486G variant-expressing cells in patient GOE615 in red and the regulon for variant-dependent genes. The heatmap displays the scaled, normalized gene expressions of the top 30 markers with highest adjusted p values from the regression of the gene expressions to the mutation rate (p values shown in top bar), and a bar graph on the left shows mutant cell fraction in each cluster labelled to the left of the heatmap. (c) t-SNE projection showing non-uniformly distributed MT-CYBL236I variant-expressing cells in GOE1360 in red and the regulon for variant-dependent genes, with a bar graph showing mutant cell fraction in each cluster labelled to the left of the heatmap.
Licensed under: https://creativecommons.org/licenses/by/4.0/